https://doi.org/10.25237/congreso2023-6 | PDF
Rodrigo Gutierrez Rojas1, Ran Liu2, Rory Mather2, Tom Stone2, Laura Santa Cruz2, Edward Bittner3, Patrick Purdon Gutierrez2
1University of Chile
2Harvard University
3Massachussetts General Hospital
Introducción
Preoperative knowledge of expected postoperative pain can help guide perioperative pain management and focus interventions on patients with the greatest risk of acute pain. However, current methods for predicting postoperative pain require patient and clinician input or laborious manual chart review and often do not achieve sufficient performance (1).
Objetivos
To develop a machine learning method able to predict maximum pain scores on the day of surgery and four subsequent days and validate this method in a prospective cohort
Materiales y Métodos
The stuy protocol was approved bu the local Institutional Review Board (# 2020P000301 ) and the requirement for consent form was waived. We included adult patients undergoing non cardiac surgery with general anesthesia. Data was obtained directly from the electronic health record (EHR). This is a secondary analysis of a previous work already published (2).
Our model consists of a neural network comprised of an embedding layer, multi-head self-attention layer (3), and a densely connected feed-forward network. For each patient, the network takes as input their set of current procedure terminology (CPT) and International Statistical Classification of Diseases (ICD-10) codes, and computes a 256-dimensional vector, which we refer to as the set embedding. This set embedding is concatenated with a vector of demographic and preoperative variables and is then passed to the feed-forward network, which predicts the maximum pain score on the day of surgery and four subsequent postoperative days (using the Numeric Rating Scale, NRS). We developed the prediction model using a multicenter retrospective dataset, and prospectively evaluated its performance. We also collected clinician predictions of postoperative pain for surgical cases in the prospective cohort and compared the performance of our model’s predictions against clinician predictions.
Resultados
In our retrospective cohort (n=234.274 from 4 hospitals) , our model achieved moderate performance in predicting moderate (Figure 1A, defined as a maximal pain score above 4) and severe pain (Figure 1B, NRS >6). Area under the receiver operating curve (AUC) ranged between 0.73 and 0.79 on postoperative days 0 through 4 for predictions of moderate pain, and between 0.72 and 0.76 for predictions of severe pain. In our prospective cohort, the model predicted postoperative pain with performance comparable to that achieved in the retrospective data, though with poorer performance on postoperative days 0 and 1. AUCs ranged from 0.67 to 0.76 on postoperative days 0 through 4 for predictions of moderate pain (Figure 2A-E), and between 0.64 and 0.79 (Figure 2F-3J) for predictions of severe pain. Remarkably, our model ouperformed clinicias predictions (Figure 2).
Conclusiones y/o implicaciones
Our method, called POPS, is fully automated and relies only on data available prior to surgery obtained directly from the EHR, allowing application in all patients scheduled for or considering surgery. POPS achieves state-of-the-art performance and outperforms clinician predictions on all postoperative days when predicting maximum pain on the 0-10 NRS in prospective validation, though with degraded calibration. POPS is interpretable, identifying comorbidities that significantly contribute to postoperative pain based on patient-specific context, which can assist clinicians in mitigating cases of acute pain.
Referencias
1. Joshi, GP, Ogunnaike, BO. Consequences of Inadequate Postoperative Pain Relief and Chronic Persistent Postoperative Pain. Anesthesiology Clinics of North America 2005, 23:21-36. https://doi.org/10.1016/j.atc.2004.11.013
2. Santa Cruz Mercado, LA, Liu R, Bharadwaj K, Johnson J, Gutierrez R, Das P, et al. Association of Intraoperative Opioid Administration With Postoperative Pain and Opioid Use. JAMA Surgery 2023, in press. https://doi.org/10.1001/jamasurg.2023.2009
3. Ilse, M, Tomczak, J,Welling, M. Attention-based Deep Multiple Instance Learning. in Proceedings of the 35th International Conference on Machine Learning 2018; 2127-2136.
Tablas e Imágenes
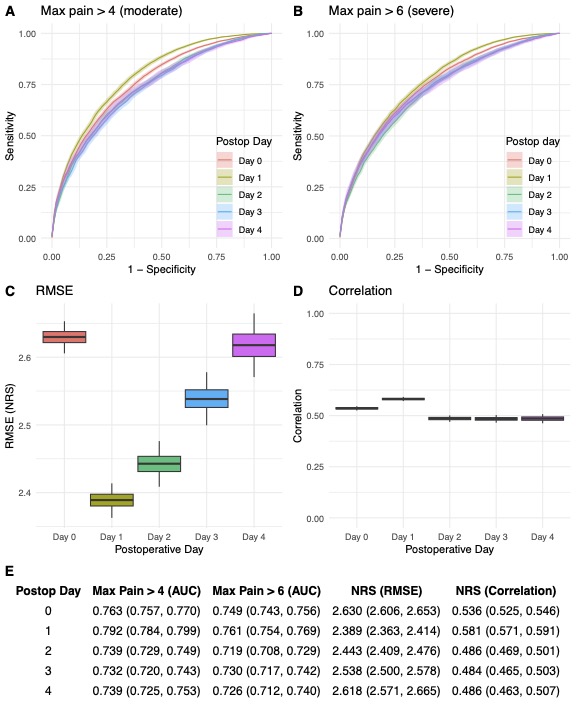
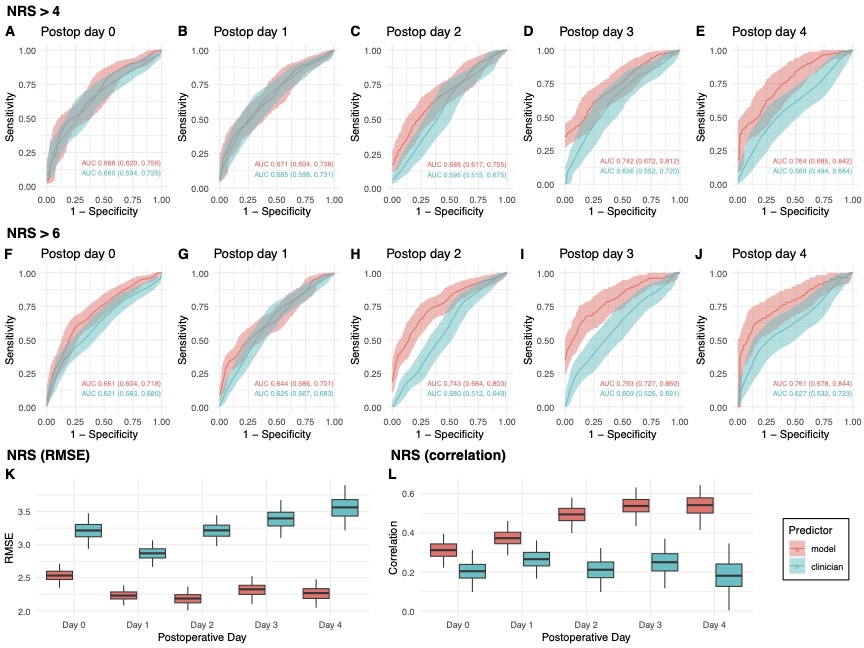