https://doi.org/10.25237/congreso-2022-15 | PDF
Catalina Saini (1), Carlos Valle (1), Gabriela Vargas (1), María Rodríguez Fernández (1)
Ignacio Cortínez (1), Ranganatha Sitaram (2).
-
Pontificia Universidad Católica de Chile
-
St. Jude Children’s Research Hospital
Introducción
Current evaluations of consciousness during anesthesia have limited precision. This can produce negative clinical consequences such as intraoperative awareness or neurological damage due to under- or over-dosage of anesthesia, respectively. Activity in frontal and parietal areas has been correlated to consciousness, including the modulation of consciousness through anesthetics.
Objetivo General
This study aims to assess consciousness in wakefulness, deep sedation, and intermediate levels through biomarkers of electrical and hemodynamical brain activity.
Material y Métodos
Volunteering patients undergoing an endoscopy or colonoscopy procedure are monitored during a stepped propofol infusion. We use a synchronized hybrid measurement system combining frontal and parietal electroencephalography (EEG) and parietal functional near-infrared spectroscopy (fNIRS). Five consciousness levels are defined using the Modified Ramsay Sedation Scale (MRSS) based on the patient’s response to verbal, motor or painful stimuli.
The frequency spectrum bands and the oxygenated (HbO) and deoxygenated hemoglobin (HbR) fluxes are obtained from the EEG and fNIRS signals, respectively. In order to develop a machine learning algorithm capable of decoding levels of consciousness, the signals are divided into epochs of 8 seconds after the onset of each condition. From each epoch, the theta, alpha and beta frequency bands in EEG, and mean, peak and variance HbO and HbR in fNIRS, are used as features. These features are given to a supervised classifier, together with their assigned level of the MRSS.
Resultados
As preliminary work, LDA classification models were trained with the parietal data of three subjects considering two conditions: resting state (baseline) and loss of consciousness (MRSS level 5). Two models were developed: EEG only and fNIRS only. Our preliminary models perform well, obtaining an F1 score of almost one when using EEG and significantly lower (near 0.7) for fNIRS. Both classifiers reached best performance using around 20 epochs from the trigger onset. As the number of epochs after the onset of the condition increases, the performance of the classifier decreases. The performance loss is abrupt after 80 and 50 epochs for EEG and fNIRS, respectively.
Conclusiones y/o Implicaciones
Anesthesiologists will benefit from the complementary information for better anesthesia induction. This can help prevent negative clinical consequences such as anesthesia overdosage and intraoperative awareness.
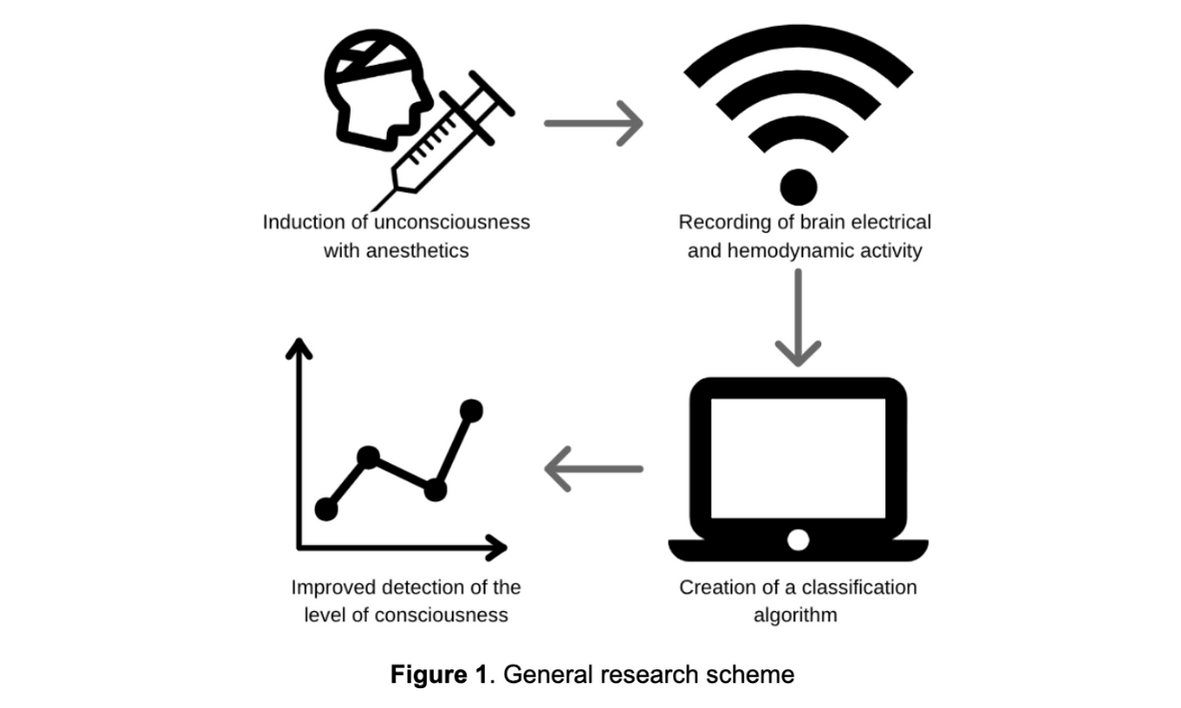
Figura 1
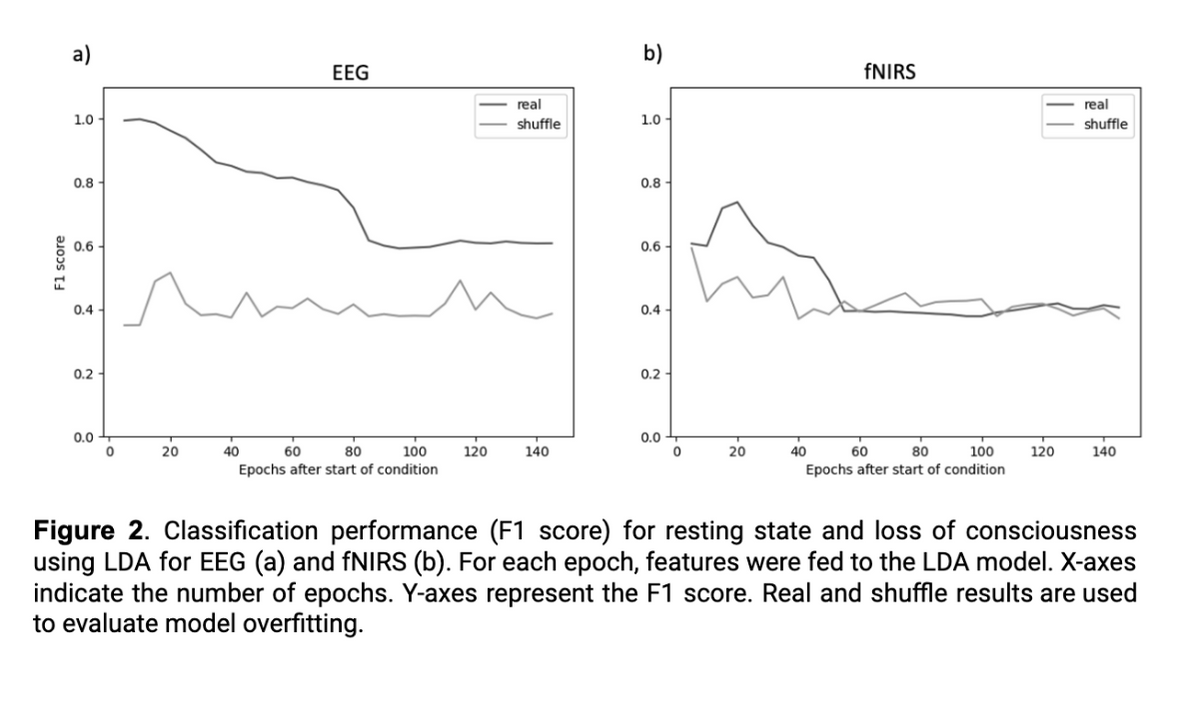